The Evolution of Competitive Rocket League Through the Lens of RLCS League Play
Rocket League gameplay has evolved greatly over the RLCS seasons: from its infancy in Season 1 when the air dribble was a new mechanic, to the current high-flying demo-heavy meta.
How can this progress be broken down?
The stages of motor learning 6, can quite cleanly be applied to describe the various seasons.
In the following, the League Play stages of each season are described through the average stats of all players that took part (in NA and EU).
Statistics Methodology
Feel free to skip this part if it does not interest you. It is not important to the narrative.
A long list of stats was calculated for each player in every replay available from the League Play stages. The average over all the player’s games from that season was then used for each stat.
These stats were then fed into a factor analysis procedure. This analysis indicated that >80% of the variance in the stats could be explained by 9 factors; each factor is a linear combination of all stats. While these algorithmically generated factors (Sheet 1: Factor Loadings From Factor Analysis) were not used in the following analysis, it informed the creation of the 9 factors, each with hand-picked weights for each stat. These curated factor weights (Sheet 2: Curated Factor Loadings) result in more interpretable factors as each factor was now a weighted sum of only a few chosen stats.
Each player’s stats averages were then summed according to the relevant weights to result in a description through these 9 factors:
- Speed & Boost Usage
- Attacking Positioning
- Average Boost Level
- Aerial Affinity
- Dribbling & Wall Hits
- Passing
- Shot:Save Ratio
- Demos
- Big Pad Reliance
*These stages as labelled are not exclusive. Previous stages are continually revisited through exploration of new options. Because many of the little pieces of mechanics that make up Rocket League plays utilise open skills7, the cognitive and associative stages are often revisited in the learning of newly-relevant mechanics8.
Four the nine factors are visualised in the following graphic. More can be found in the Visualisations section below.
The Stages of Motor Learning
Fitts PM, Posner MI. Human performance. 1967.
Season 1 to 3 – The Cognitive Stage
“In the first stage, movements are slow, inconsistent, and inefficient, and large parts of the movement are controlled consciously.”
Weaver J. Motor learning unfolds over different timescales in distinct neural systems. PLoS biology. 2015 Dec;13(12).
https://doi.org/10.1371/journal.pbio.1002313
Season 1 was professional Rocket League at its earliest days. People were not very good at the mechanical aspects of the game, and the finals were at a low-Champ (C1-C2) level in terms of gameplay 9.
In fact, so much has changed since that the only player still relevant from that time is Jessie. Unsatisfied with guiding his team to the top 4 in RLCS S1, he has persevered in his quest for “that trophy”. In the latest season, he has surpassed the lofty bar he set in that first season by leading his team to the Grand Finals in the S8 World Championship.
This stage is characterised by a consistent increase in speed and aerials with a high reliance on big boost pads.
From an average speed of 1453 in S1, players sped up to an average speed of 1504 by S3 (for reference, maximum speed achievable with throttle is 1400, in units of uu/s). Boost usage likewise increased consistently from 1822 to 2054 and then to 2141.
The fraction of hits that were aerials increased from 16% to 23%.
Roughly constant throughout these seasons, players collected roughly 3 small boost pads per big boost pad.
*As there was no League Play stage in Season 1, the stats shown are for Qualifier 2.
Season 4 to 6 – The Associative Stage
“In the second stage, movements become more fluid, reliable, and efficient, and some parts of the movement are controlled automatically.”
Weaver J. Motor learning unfolds over different timescales in distinct neural systems. PLoS biology. 2015 Dec;13(12).
https://doi.org/10.1371/journal.pbio.1002313
As players familiarised themselves with the basic mechanics, the speed of the game plateaued.
Gross movements become refined, and anticipation becomes a bigger part of the game. This is reflected by the Gale Force eSports/Dignitas roster that dominated this era having middling speed and boost usage stats. (While ViolentPanda is among the forefront in terms of speeds and aerials in S3 and S4, he moves further away from the front across S4-S6)
Seasons 4 to 6 saw a stagnation in speed, with average speed changing from 1525 to 1535 and then back down to 1522. Boost usage decreased between these seasons, from a high of 2209 in S4 to 2200 in S5 and 2187 in S6.
The number of aerials however kept rising, increasing to over 26% of all hits.
As players started noticing the importance of pathing over small boost pads, the number of small boost pads used increased to over 3.6 per large boost pad.
Season 7 & 8 – The Autonomous Stage
“And in the third stage, movements are accurate, consistent, and efficient, and movement is largely controlled automatically.”
Weaver J. Motor learning unfolds over different timescales in distinct neural systems. PLoS biology. 2015 Dec;13(12).
https://doi.org/10.1371/journal.pbio.1002313
As players get increasingly comfortable with the more advanced mechanics of the game, the finer aspects of various mechanics get perfected. Momentum conservation, better pathing over small boost pads, and better timing for big pad collection all reflect these subtle improvements.
The anti-game mechanic of demolitions roars to the forefront of the meta as forcing mistakes becomes a necessity.
In this stage, playstyles become more varied. The figure below shows the average spread in each factor, and the bold dark blue line indicates the average over all 9 factors. This spread can be seen to decrease from season 1 to 6, but increases in seasons 7 and 8.
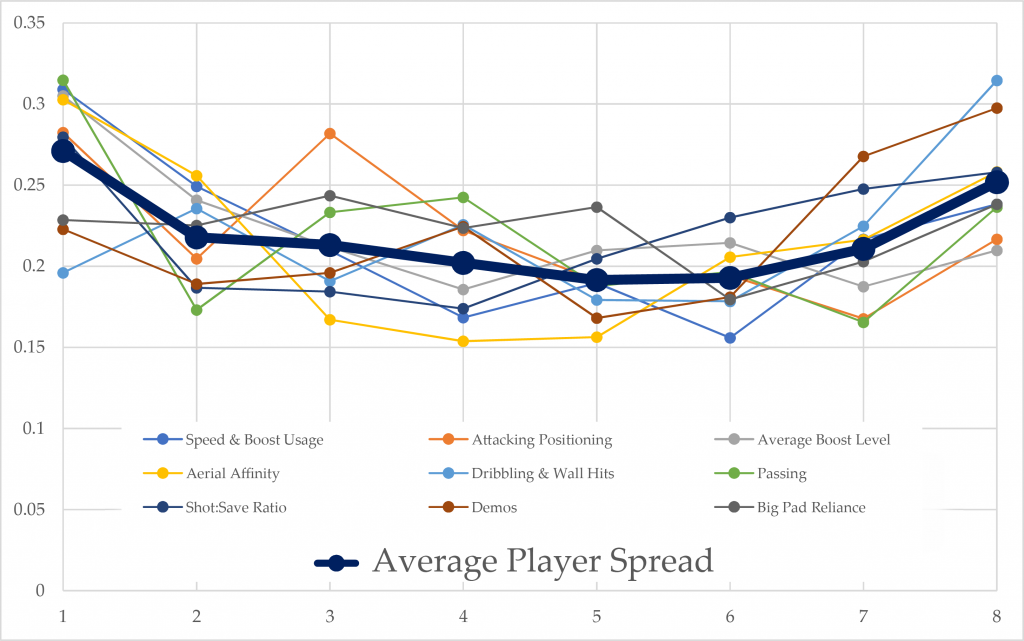
The Rise of Difference: The standard deviation of each factor across the seasons.
The average standard deviation of each factor increases from below 0.19 in seasons 5 and 6 to 0.20 in season 7 and 0.24 in season 8, indicating differences between players not seen since season 1.
The previous two seasons saw further increases in average speed, with players hitting average speeds of 1545 and then 1552. Boost usage hit all-time highs of 2216 and 2253 in these latter seasons.
The number of small pads collected per game increased by almost a third to 41.
Demos increased to an average of 2.5 per team per game in S8 from 1.8 in S5.
Visualisations
Evolution over seasons shown through pretty graphs
The following plots highlight factors that are highly correlated to the season iteration:
- Graphs:
- Factors:
- Speed & Boost Usage
- Aerial Affinity
- Dribbling & Wall Hits
- Demos
The following plots highlight factors that are not strongly correlated to the season iteration, likely highlighting playstyles/roles/strategies:
- Graphs:
- Factors:
- Attacking Positioning
- Average Boost Level
- Shot:Save Ratio
- Passing & Attacking Pressure
Other findings
Some of the research into basic Physical Education (such as the classification of skills on a open-closed spectrum) ties in neatly with the observable practice tendencies of most current RL pros.
- The open skills of Rocket League require interaction with teammates and opponents.
- Competitive play (e.g. Ranked or 6Mans), offering this dynamic gameplay, is by far the most common form of practice.
- The static practice offered by repetitive training packs are likely insufficient to advance the players’ open skills.
- Free-play training, where players can constantly interact with the ball and the stadium, is where most pros seem to hone their closed skills.
Part II
I will go through the players that were exceptional in the various stats in Part II, which can be read here.
TL;DR
- S1-3: Citius.
- S4-6: Altius.
- S7-8: Fortius.
- Check out the pretty graphs above.
- Part II is now published here.
Footnotes:
- Notes on open and closed skills: https://www.bbc.co.uk/bitesize/guides/zgtmp39/revision/3
- Article on the stages, includes examples of revisiting previous stages: https://us.humankinetics.com/blogs/excerpt/understanding-motor-learning-stages-improves-skill-instruction
- Notes on open and closed skills: https://www.bbc.co.uk/bitesize/guides/zgtmp39/revision/3
- Article on the stages, includes examples of revisiting previous stages: https://us.humankinetics.com/blogs/excerpt/understanding-motor-learning-stages-improves-skill-instruction
- Blog post estimating the rank of season 1 gameplay: http://blog.calculated.gg/2019/01/if-rlcs-season-1-players-were-transported-to-2019-what-rank-would-they-be/
- Fitts PM, Posner MI. Human performance. 1967.
- Notes on open and closed skills: https://www.bbc.co.uk/bitesize/guides/zgtmp39/revision/3
- Article on the stages, includes examples of revisiting previous stages: https://us.humankinetics.com/blogs/excerpt/understanding-motor-learning-stages-improves-skill-instruction
- Blog post estimating the rank of season 1 gameplay: http://blog.calculated.gg/2019/01/if-rlcs-season-1-players-were-transported-to-2019-what-rank-would-they-be/